NLP: The future of data management in life sciences
Research & Development
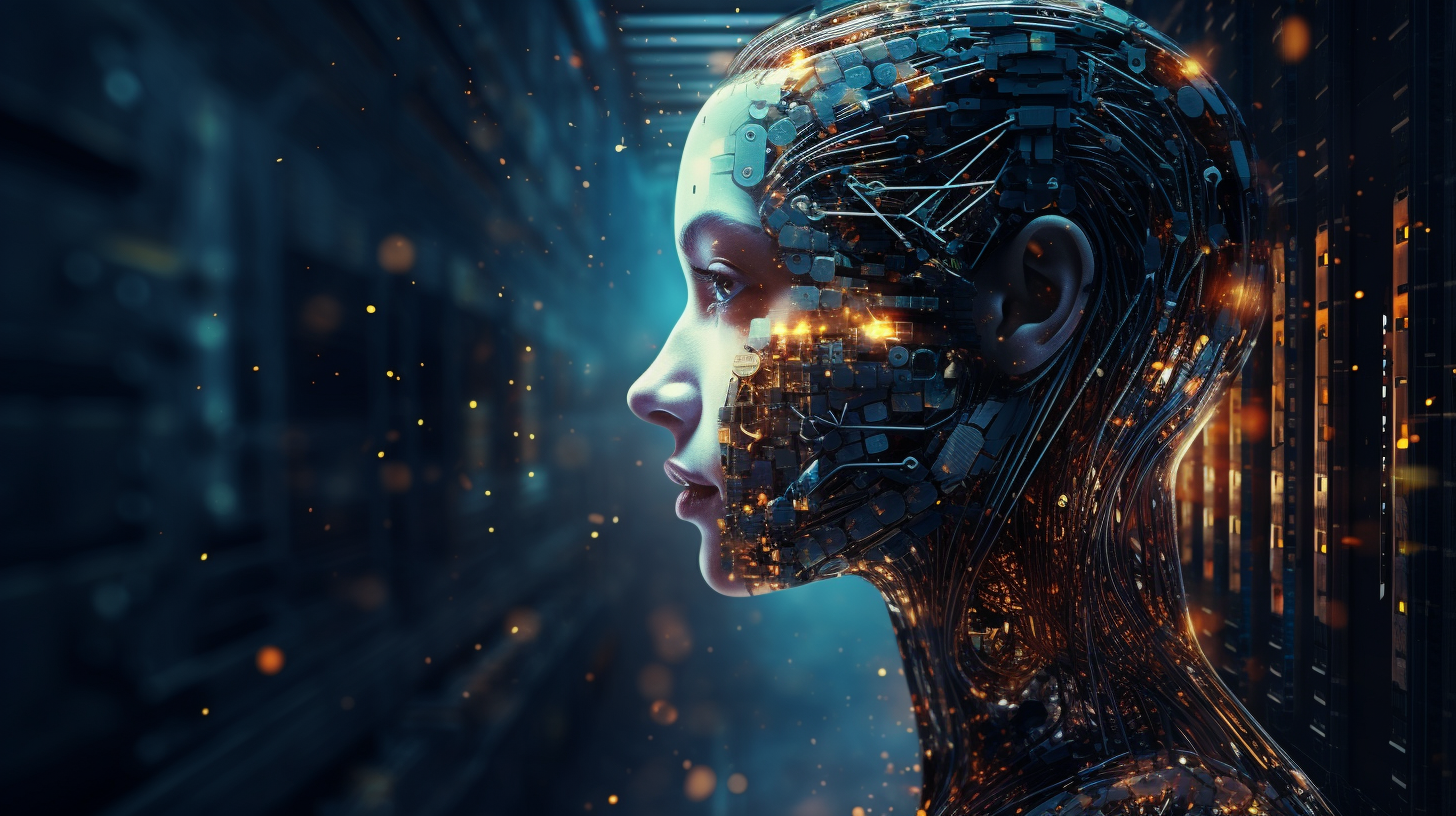;)
Imagine a world where machines understand human language as well as we do, unlocking a treasure trove of untapped data in the life sciences industry. This isn’t science fiction; it’s the transformative power of Natural Language Processing (NLP). This article is the first in a series that explores how NLP is revolutionizing data management and analysis in life sciences, offering new avenues for research and development.
What are unstructured documents?
First, let’s clarify what we mean by unstructured documents. These are files or data sets that are not easily interpretable by machines, although humans have little trouble understanding them. The category is broad and includes:
Emails
Conversations between researchers, clinicians, or various departments often contain valuable but informal insights that are not readily quantifiable.
Research papers
These documents can be complex and are not always straightforward to categorize, especially for those who are not experts in the field.
Social media posts
These platforms offer a wealth of public opinions and insights but are often overlooked as valuable data sources.
Patient records
These documents often contain both structured and unstructured data and raise concerns about compliance and data protection, especially in the era of GDPR.
Dossiers and templates
These documents encapsulate the expertise and processes of entire organizations and could be gold mines for big data.
Why information extraction matters
The extraction of data from these unstructured documents is not just a technical challenge; it’s a necessity for several reasons:
Accelerated discoveries
Mining data from archived documents can expedite research and development processes, enhancing productivity without overstretching resources.
Comprehensive analysis
Machines are particularly skilled at analyzing large sets of data and identifying correlations within them, something that would take humans significantly more time.
Scalability
NLP tools offer the ability to process large volumes of data in a time-efficient manner, making them invaluable for large-scale projects and long-term data management.
Demystifying NLP
Natural Language Processing (NLP) is a dynamic field that has undergone remarkable transformations since its inception. It serves as a bridge between human language and machine comprehension, aiming to teach computers to understand, interpret, and generate human language in a way that is both meaningful and useful. Initially, the field was dominated by rule-based methods, which were rigid and limited in scope. However, the shift towards statistical models in the late 20th century opened new avenues for understanding language patterns.
The real game-changer came with the advent of machine learning algorithms in the 2000s. These algorithms could learn from data, improving their performance as they were exposed to more and more examples. This led to the development of more sophisticated NLP applications, from chatbots to translation services. More recently, deep learning techniques have pushed the boundaries even further. Architectures like OpenAI’s GPT-3 and Google’s BERT have set new standards for what machines can understand and generate, achieving performances that are sometimes indistinguishable from human capabilities, as claimed in this article.
Interesting facts
The Turing test
Proposed by Alan Turing in 1950, this test measures a machine’s ability to exhibit human-like intelligence, as explained by the Stanford Encyclopedia of Philosophy. It’s often considered the gold standard for evaluating AI conversational capabilities.
Data-driven
The advancement of NLP owes much to the availability of extensive online data, which has been a game-changer in training algorithms. For example, Google’s BERT was trained on BooksCorpus (800 million words) and the English Wikipedia (2,500 million words).
Multilingual models
Recent developments have produced NLP models capable of understanding multiple languages, sometimes without explicit training, as discussed in this study from researchers at Cornell University.
Leveraging NLP in life sciences
In the life sciences industry, NLP can offer various benefits:
Contextual analysis
NLP can discern between different medical conditions described in a patient's narrative, such as a common cold and an allergic reaction.
Sentiment detection
In drug trials, participant feedback is vital. NLP can sort this feedback into positive, neutral, or negative sentiments, providing a more nuanced understanding of trial results.
Relationship extraction
NLP can identify overlaps in research, revealing potential interdisciplinary insights that could lead to groundbreaking discoveries.
Regulatory task enhancement
NLP and Generative AI advancements can streamline data extraction, making it easier to comply with various regulations and guidelines.
Summarization and text generation
By leveraging LLMs and NLP, we can accelerate text summarization, efficiently extract pertinent information from extensive documents, and even generate new content.
Conclusion
Natural Language Processing (NLP) stands at the forefront of AI research and has the potential to redefine the future of human-machine interactions. Its application in the life sciences sector can unlock a wealth of often-underutilized data, thereby refining research and development processes and leading to more effective and efficient outcomes.
Get in touch
If you’re as captivated by the transformative potential of life sciences as we are, why not reach out? We’re always eager to discuss how we can collaboratively harness the power of innovation to achieve your goals.